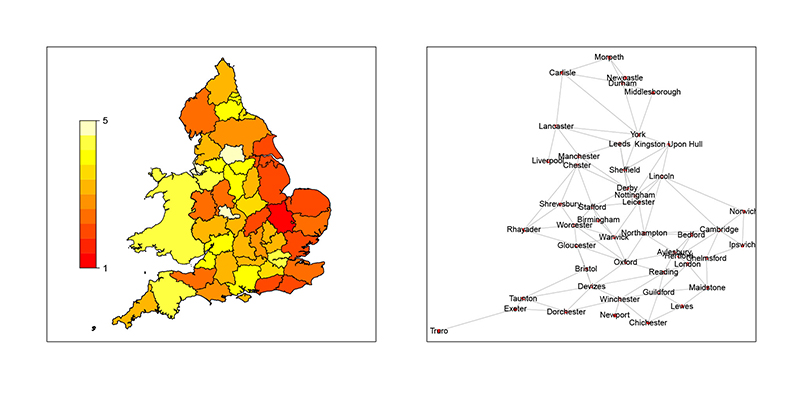
Network data
Stochastic processes recorded on networks (graphs) are rich data objects with overlapping layers of potentially interacting information.
Network data has recently known an explosive expansion, arising across a multitude of fields from biology and medicine, to transportation and cyber security, reverberating at the level of the environment and climate.
With the increasing volume of network data, their nature and collection have also become increasingly complex. For example, we now have access to large dynamic networks in continuous or discrete time – network time series – where the underlying graph structure and/or distribution may be static or changing, the process may exhibit nonstationarity in time or space, vector or matrix values may be recorded on edges and/or nodes. Naturally, we may observe collections of network time series that are intrinsically related to one another. Take for example plants’ response to environmental stress as quantified by gene (nodes) co-expression (edges) in a graph whose dynamic structure we aim to model and ultimately forecast.
Driven by such complex objects, unsurprisingly the answers to the research questions posed by their analysis either currently do not exist or are in development stages. Proof to the pressing nature of achieving a step change in the modelling and prediction of vast banks of ever-growing, interconnected network data is the strategic EPSRC investment in a programme grant that harnesses statistical and probabilistic expertise across six UK universities. Statistics at York is one of its nodes (more information is at NeST), with investigations focusing around the use of multi-scale second generation wavelet decompositions for network inference, including for properties such as long memory.