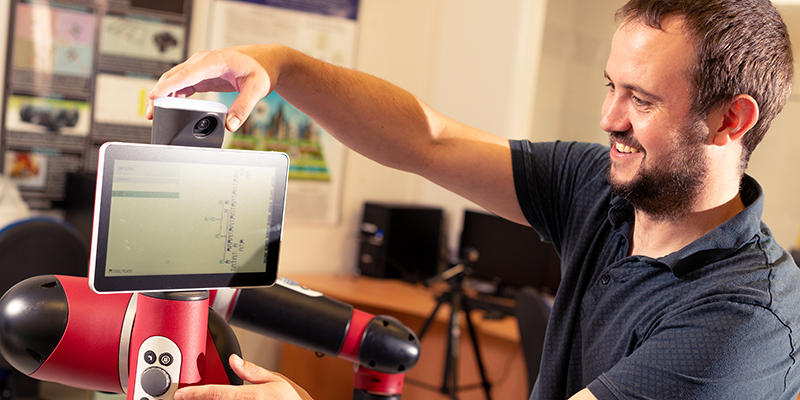
Centre for Doctoral Training in the area of Autonomous Robotic Systems for Laboratory Experiments (Albert CDT) - Funded PhD Study
Apply for a fully funded project in the YorRobots ALBERT CDT.
YorRobots is proud to announce the launch of its Centre for Doctoral Training in the area of Autonomous Robotic Systems for Laboratory Experiments (ALBERT CDT).
Join us and contribute to the development of the science, engineering and socio-technology that underpins autonomous robotics for chemistry, computer science and related sciences.
Explore our opportunities spanning a range of topics relating to autonomous robotics and their uses. We have fully funded projects available for the September 2024 start.
Extended deadline for applications: 17:00 GMT on Wednesday 31 January 2024
Funding
Our PhD opportunities are fully funded for up to 3.5 years by the Engineering and Physical Sciences Research Council (EPSRC) and the University of York.
As a postgraduate researcher with the ALBERT CDT, you'll receive the following financial benefits:
- A tax-free annual stipend at the standard Research Council rate (£18,622 for 2024-25, and pro rata for part-time PhD researchers). This will be paid to you in regular instalments. The amount of the stipend usually increases each year in line with inflation
- Your annual tuition fees will be paid.
- There will be funding available to you for attendance at relevant events and conferences, and for project-related consumables.
Due to funding arrangements, the number of opportunities available to international applicants is limited; however, we encourage you to still apply.
Please be aware that funded PhD opportunities in the ALBERT Centre for Doctoral Training are not available via distance learning. If you need a Student Visa in order to study in the UK, please note that you must study as a full-time student. It is not possible for you to study on a part-time basis.
What to know
Diverse Topics
We offer a wide range of funded PhD projects, from understanding chemistry labs for automation to designing robot parts.
Entry Requirements
You should have, or expect to obtain, a Bachelor's degree with a 2:1 (or equivalent) in any relevant discipline. Please check the listing for the project you are interested in.
Apply
It's as easy as filling in the application form and listing us as the source of funding. No project proposal section is needed.
Entry requirements
Knowledge and experience
You should have, or expect to obtain, the equivalent of a UK upper second class (2:1) degree in a relevant discipline for the project for which you are applying. This is the University’s standard requirement, and applicants are advised to check the individual requirements for each project listed.
English language requirements for international students
If English is not your first language, you must provide evidence of your ability. On the University's English Language requirements web page, you can find out the grades each department requires, and which tests we accept.
Read more about English Language requirements for research degrees
Course location
You'll be based in the buildings of the lead department for your project at the University of York.
Our two lakeside campuses are exciting and welcoming hubs for innovation and collaboration, with world-class laboratories and modern working spaces. You'll benefit from the CDT's multidisciplinary approach, working across the disciplines of computer science, chemistry, engineering, psychology, sociology and law.
Most of your training and supervision meetings will take place on campus at the University of York, though your research may take you further afield.
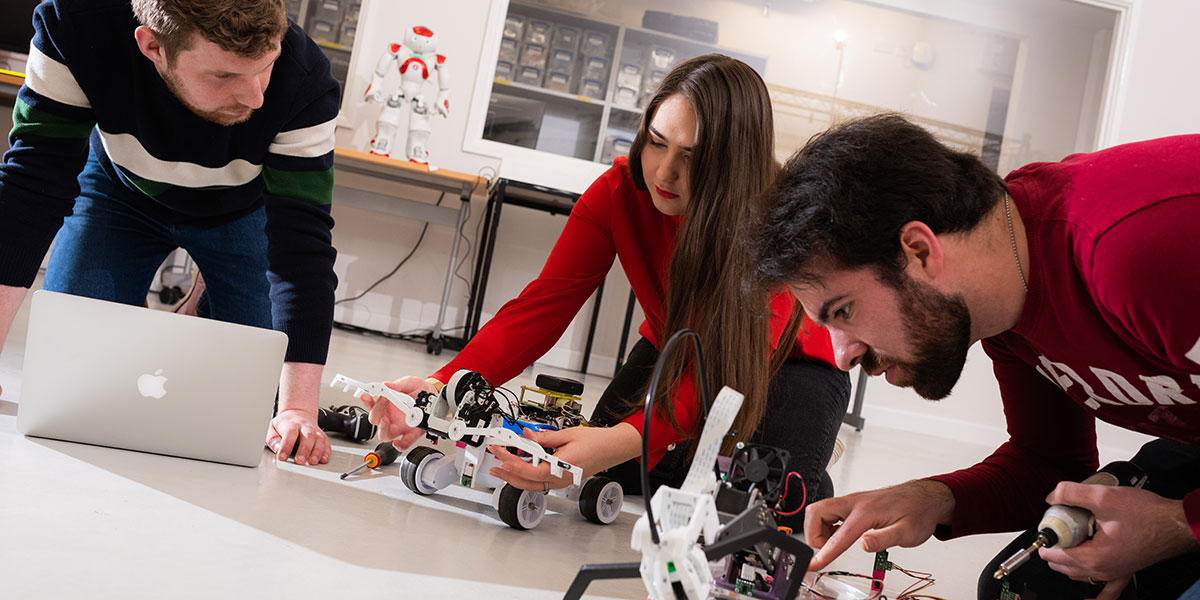
Find Out More
Video PhD Project Descriptions
In this video, find out more about our wide range of PhD projects relating to autonomous robotics and their uses. Each speaker will outline their project's aims and objectives and will describe the opportunities for PhD study in their area.
Click through to watch the video on YouTube to see each project section in the video.
Funded projects
Explore our opportunities spanning a range of topics relating to autonomous robotics and their uses.
If you have any questions about individual projects, please email the lead department's postgraduate admissions team. You will find the contact email address on the departments' admissions web pages.
How to apply
- Select your project from the section below and click the 'Apply for this project' button.
- You will be taken through to the postgraduate admissions web page for the department that is leading the project you are interested in. Here, you will see a link to the University's online application form for postgraduate study.
- When you complete the online application form, please select 'CDT Autonomous Robotic Systems for Lab Experiments' as your source of funding. You do not need to provide a research proposal: just enter the name of the project you wish to apply for.
School of Physics, Engineering and Technology
Professor Andy Tyrell, Professor Ian Fairlamb and Dr Darren Reed
Robots are used in many applications. A number of these require grasping in one form or another. These tend to be designed for one application and “hand-designed”. A more generic approach using evolutionary methods should be able to produce grasping mechanism suitable for many applications.
The proposed project’s focus will be on autonomous execution of robotic grasping tasks, and the incorporation of evolutionary methods for on-line adaptation, both in terms of evolving the grasping mechanism(s) and evolving the robot’s behaviour and learning process. The work will involve a number of novel areas, consisting of experimental work as well as simulations: (i) Applying evolutionary methods on robotic grasping tasks on-line, using sensory data gathered from realistic tasks and then (ii) Optimising the capabilities of a robotic gripper to execute a given task in terms of hardware, intelligence, and constraint fulfilment.
The project will provide excellent opportunities for training in the areas of robotics, evolutionary computing and experimental laboratory practice.
Department of Computer Science
Professor Dimitris Kolovos and Dr Simos Gerasimou
Scientific experiments are an essential part of scientific research, as they allow scientists to test hypotheses, collect data, and validate theories. However, designing and executing experiments can be a complex and error-prone task, especially when dealing with large, complex, and dynamic systems that involve collaboration between humans and robots, such as those found in modern laboratories. Traditional approaches to experiment design and execution rely on ad-hoc solutions, such as handwritten protocols, spreadsheets, and custom software, which can be difficult to understand, maintain, and share.
Domain-Specific Languages (DSLs) [1] offer a promising alternative to these approaches, as they allow domain experts to specify experiment designs and protocols [2] using a specialised, concise, and intuitive language that is tailored to their specific needs and requirements. DSLs can be used to encode the details of an experiment, such as the materials, equipment, procedures, and measurements, in a structured and formal way that can be validated, simulated, and executed. DSLs can also provide support for experiment design and analysis, such as visualization, optimization, and verification, by leveraging domain-specific knowledge and techniques.
The project will address the following research questions:
- What types of DSLs (e.g. graphical, textual, projectional, hybrid) are most suitable to support the specification of scientific experiments that require collaboration between robots and humans (e.g. scientists, technicians) in laboratory environments?
- How can DSLs be integrated with existing experiment design and execution processes and platforms to provide a seamless and efficient experience for different stakeholders?
- How can the effectiveness and efficiency of using DSLs for scientific experiment design and execution be evaluated and compared to traditional approaches, in terms of productivity, reproducibility and collaboration?
In the context of this project the student will:
- review literature on domain-specific language engineering and on human-robot collaboration
- become familiar with state-of-the-art technologies for domain-specific language development and model management
- conduct interviews and surveys with domain experts, such as scientists and laboratory technicians, to gather requirements and feedback on the use of DSLs for experiment design and execution
- design and implement DSLs for scientific experiment design and execution, based on the identified requirements and best practices
- integrate the DSLs with existing experiment design and execution processes and platforms, and evaluate their effectiveness and efficiency through case studies, experiments, and user feedback.
Novelty
The project will produce novel methods for designing and implementing domain-specific languages and supporting tools for collaborative human-robot scientific experiments.
References
[1] Arie van Deursen, Paul Klint, and Joost Visser. 2000. Domain-specific languages: an annotated bibliography. SIGPLAN Not. 35, 6 (June 2000), 26–36. https://doi.org/10.1145/352029.352035
[2] Silva, E., Leite, A., Alves, V. et al. ExpRunA : a domain-specific approach for technology- oriented experiments. Softw Syst Model 19, 493–526 (2020). https://doi.org/10.1007/s10270-019-00749-6
[3] Ajoudani, A., Zanchettin, A.M., Ivaldi, S. et al. Progress and prospects of the human–robot collaboration. Auton Robot 42, 957–975 (2018). https://doi.org/10.1007/s10514-017-9677-2
Department of Chemistry
Dr Charlotte Willans, Professor Ian Fairlamb and Dr Laurence Wilson
Organometallic catalysis is one of the most vibrant and essential areas worldwide in scientific research, with impact in a broad range of industrially relevant fields such as pharmaceuticals, agrochemicals and materials. Many metal-catalysed reactions rely on the use of precious metals such as palladium, iridium and rhodium; the high cost of these metals and risk of dwindling supply render these processes unsustainable. Attention over the last decade has turned towards the development of more abundant and cheaper basemetals. Major challenges in this field are a lack of understanding and low predictability, thus significantly higher loadings of catalyst are used when compared to precious metals. Despite being more abundant and cheaper, many of the base metals pose significant toxic risks, both when handling the precursors prior to the reaction and in their disposal, particularly as high loadings are used. Nickel, which has been developed as a highly attractive alternative for many metal-catalysed processes,1 is highly toxic.
This project will explore the use of robotics to reduce and ultimately eliminate the toxic effects of nickel when used in processes relevant to the pharmaceutical and agrochemical sectors. The generation of (pre)catalysts electrochemically and in flow,2 directly from a metal plate, will eliminate the need to handle nickel precursors in the form of powders prior to a reaction. The use of a unique flow platform for catalyst screening and optimisation3 will generate data to understand the processes, thus enabling the amount of nickel required for a particular reaction to be reduced. Electrochemical recovery of the nickel following the process will reduce the risk of the metals being released into waste streams. The ultimate goal is to have a closed loop system (see Figure) whereby the catalyst is electrochemically generated from a nickel plate, used directly in a catalytic reaction (either for screening or for large-scale industrial synthesis), and the nickel electrochemically removed back on to a plate and looped back for reuse. The technological development will also be relevant to other types of metal catalysts and processes.
- Ananikov VP. Nickel: the “spirited horse” of transition metal catalysis. ACS Catalysis 2015, 5, 1964-
1971. - Chapman MR, Shafi YM, Kapur N, Nguyen BN, Willans CE. Electrochemical flow-reactor for
expedient synthesis of copper-N-heterocyclic carbene complexes. Chemical Communications 2015,
51, 1282-1284. - Schotten C, Manson J, Chamberlain TW, Bourne RA, Nguyen BN, Kapur N, Willans CE. Development
of a multistep, electrochemical flow platform for automated catalyst screening. Catalysis Science &
Technology 2022, 12, 4266-4272.
Department of Psychology
Dr Cade McCall, Professor Ana Cavalcanti and Professor Jason Lynam
Human decision-making is critical for the safety and efficacy of supervised autonomous systems. As such, human behaviour should be accounted for in their design and verification. But modelling human decision-making is not trivial. Humans deploy a wide variety of cognitive processes to make decisions in the complex, dynamic and unpredictable environments in which we live and work. We use prior knowledge, attend to ongoing changes in context, reason about the likely outcomes of possible choices, and weigh their costs and benefits. But key questions arise with the introduction of autonomous systems to living and working environments. Does sharing a task with an autonomous system shape our approach to dealing with uncertainty, our attention to key contextual factors, our willingness to take risks, or our ability to learn through trial and error? Addressing questions such as these will help us anticipate how autonomous systems shape our cognition in situations where human input is critical but potentially fallible. Moreover, answering them will help us incorporate accurate models of human behaviour when designing safe and effective autonomous systems. The proposed project will explore these ideas with specific reference to autonomous systems designed for use in chemistry labs. It will examine how such systems can support the decision-making involved in a laboratory’s data acquisition and analysis, while identifying more general principles for optimizing human-robot interaction.
- Identify key parameters of human decision-making that are likely to be affected by collaborative work with autonomous systems in laboratory environments. These
parameters will be drawn from the extant decision-making literature, making use of recent advances in cognitive modelling of dynamic decision-making. - Develop simulations in which human users complete dynamic decision-making tasks with, and without, the collaboration of an autonomous system. These simulations
will be based on existing human decision-making research paradigms that lend themselves to collaborative (i.e., human and autonomous system) decision-making. They will simulate chemistry laboratory environments in which users are tasked with data acquisition and analysis. - Gather and analyse human behavioural data using the paradigms developed in Objective 2. Analyses will employ contemporary approaches to cognitive modelling (e.g., reinforcement learning, instance-based learning, etc.).
- Provide recommendations for key factors to consider when modelling “humans in the loop” in designs for laboratory-based autonomous systems.
This project will take an empirical, quantitative approach to addressing its questions. It will rely upon human behavioural data collected in laboratory-based studies. These experiments will use immersive or desktop simulations in which individuals complete a series of decisions in a dynamic virtual environment. Data analyses will use cognitive modelling approaches to extract relevant parameters from the data and multilevel models to evaluate differences between key experimental conditions.
This research will apply contemporary approaches to measuring and modelling human decision-making to the unique contexts of human collaborations with laboratory-based autonomous systems.
- Alongside PhD research, the successful candidate will have access to training opportunities to enhance research skills and broaden their knowledge of psychology.
First year modules include modules on research design and statistics as well as practical skills in experimental, cognitive, and social psychology. Weekly research seminars in the Department of Psychology include a ‘meet the speaker' event where one can talk informally with high-profile academics and other external speakers. - The successful candidate will also be part of a cohort of our Centre for Doctoral Training on Autonomous Robotic Systems for Laboratory Experiments. This will involve
regular meetings with students from other disciplines working on similar topics. - The successful candidate will further benefit from the general training provided by the Departments of Computer Science and Chemistry. This will cover topics such as
security, research management and leadership, collaborations, employability, public engagement and communication.
References
Gonzalez, C. (2017). 13 Decision-Making: A Cognitive Science Perspective. The Oxford handbook of cognitive science, 249.
McCall, C., Schofield, G., Halgarth, D., Blyth, G., Laycock, A., & Palombo, D. J. (2022). The underwood project: A virtual environment for eliciting ambiguous threat. Behavior research methods, 1-16.
Department of Computer Science
Professor Ana Cavalcanti and Professor Ian Fairlamb
Advances in mechanics, electronics, computer vision, and artificial intelligence have recently enabled the development of exciting new robotic systems, varying from driverless vehicles to home assistants and industrial robots. In this project, we consider robots for use in Chemistry Laboratories, in particular with widely used equipment: fume cupboards. Fume cupboards are extensively used in a range of experiments. Use of these cupboards can cause physical strain, and so the amount of time that a human can operate a cupboard. To allow a robot to operate a fume cupboard, however, we need to trust that robot. Potential consequences if they fail in their operation can range from wasted time and materials, to more serious incidents involving dangerous chemicals.
In this project, we will apply state of the art technology for modelling, simulation, and testing for analysis and verification of robots that can be used with fume cupboards. We will consider, first, existing technology to evaluate the limits of what can be achieved with existing robots and existing Software Engineering techniques. Second, we will consider advanced designs that support adaptation to deal with a variety of experiments and unexpected changes to the cupboard. Contributions of the work will span from improvements to automation of a Chemistry Laboratory to novel techniques for modelling, design, and verification of adaptive software for robotics. Our challenge is to enable and promote development approaches that provide evidence that the robot can be trusted to work in the lab, dealing with sensitive materials and experiments, and around humans.
The successful candidate will benefit from the general training provided by the Departments of Computer Science and Chemistry. This will cover topics such as security, research management and leadership, collaborations, employability, public engagement and communication. Moreover, the candidate will benefit from inclusion in a cohort for our Centre for Doctoral Training on Autonomous Robotic Systems for Laboratory Experiments. This will involve regular meetings with students working on similar topics and the same case studies.
References
A. L. C. Cavalcanti, W. Barnett, J. Baxter, G. Carvalho, M. C. Filho, A. Miyazawa, P. Ribeiro, and A. C. A. Sampaio. RoboStar Technology: A Roboticist's Toolbox for Combined Proof, Simulation, and Testing, pages 249--293. Springer International Publishing, 2021. [ bib | DOI | .pdf ]
A. L. C. Cavalcanti. RoboStar Modelling Stack: Tackling the Reality Gap. In 1st International Workshop on Verification of Autonomous & Robotic Systems, VARS 2021. Association for Computing Machinery, 2021. [ bib | DOI ]
A comprehensive list of publications is provided on the RoboStar site.
Department of Computer Science
Professor Radu Calinescu and Professor Helen Sneddon
Many compounds used in industry and research labs are obtained through complex multi- stage chemical processes whose design requires the selection of suitable reactants and chemical reaction parameters such as reactant concentrations, temperature and pressure. This selection involves the exploration of very large design spaces, and can have significant safety and sustainability implications. The mathematical modelling of such processes as chemical reaction networks enables the analysis and comparison of their design options.
The project will tackle the challenging problem of synthesising chemical reaction networks (CRNs) for building user-specified chemical compounds safely and with a low carbon footprint. The project will model CRNs as continuous-time Markov chains and/or ordinary differential equations, assemble a repository of patterns of CRN fragments annotated with safety concerns and carbon-footprint levels, and use metaheuristics to instantiate and combine these patterns into end-to-end CRNs guaranteed to produce the required chemical compounds safely and with a low carbon footprint.
In a first stage, the project will model and analyse chemical processes proposed by industrial partners, identify opportunities for calibrating these processes for safety and sustainability, and validate the calibrated processes with these partners. Next, the findings from the first stage will be generalised into a methodology for the synthesis of green and safe chemical reaction networks, and the methodology will be validated through application to additional chemical processes.
While chemical reaction networks have been widely used for the design, analysis and calibration of complex chemical processes, their potential to support the synthesis of
chemical processes that are both safe and sustainable remains underexplored.
The PhD student will attend relevant graduate-level modules in the Departments of Chemistry and Computer Science, undertake visits at industrial partners, and participate in the wide range of training activities organised by our York Graduate Research School.
York Law School
Professor T.T Arvind and Dr Darren Reed and Professor Ian Fairlamb
The Albert Centre for Doctoral Training is concerned with the design of interactive robotics for the Chemistry Laboratory. As part of these efforts, we are looking for a talented lawyer with a sound background in regulation and empirical socio-legal studies to carry out research into the relationship between rules, practices, and regulation, and into how abstract policies are translated into practical processes and practices in the context of scientific laboratories. The work you will carry out will include understanding the complex relationship between the regulatory world of policy and the experiential world of practice.
You will have a fascination for human interaction with law and regulation and the personal and organisational practices through which people working in scientific contexts navigate the mesh of rules applicable to them within the laboratory space; and the processes through which they construct the relationship between rule, conduct, and space. You will also be interested in the way that embodied human interaction is shaped by their understanding of the scientific mission they see themselves as pursuing, their views as to the world of law, and the organisational cultures in which they are embedded.
You will have expertise in regulation studies and an understanding of empirical socio-legal studies. You will have a working knowledge of law and technology and/or science and technology studies, and an ability to carry out and disseminate research in a timely and constructive manner.
The principal motivation for the ALBERT mini-CDT is to develop the science, engineering, and socio-technology that underpins the building of a laboratory-based robotic system for use in applied experiments across the physical sciences. Creating a Chemistry-based eco-system that is cleaner, greener, safer, and cheaper than anything achievable by current conventional techniques and technologies, is a key driver for this research.
This research will study the multifaceted relationship between the regulatory world of policy and the experiential world of practice in the specific context of the chemistry laboratory. It will:
- study the manner in which the regulations driving the chemistry lab are instantiated at a practical and interpersonal level within, and through, everyday behaviour in the laboratory
- identify the role and impact of relational and organisational hierarchies of control, the emergence of organizational and disciplinary cultures through the communication of rules and practices via instruction and implicitly through practice, the material and textual instantiations of rules and procedures, and the establishing of habitual behaviours and practices
- develop a detailed understanding of the manner in which these behaviours and practices interact with regulatory goals, procedures, and structures to provoke questions of adherence and responsibility
- develop a detailed account of the practical instantiation of regulation in practice as instituted, socio-technical process
- draw on your findings to provide clear design requirements for other members of the Albert CDT.
The legal researcher will undertake a focused study of the development of compliance-oriented and compliance-informed processes, procedures, practices and cultures in
chemistry laboratory. The purpose of the study will be to identify the manner in which regulatory goals and policies are translated into concrete processes and practices, and the cultures of responsibility and adherence to which they give rise. Your work will be informed by a rich and nuanced engagement with regulatory theory, which you will combine with empirical socio-legally informed analysis of a laboratory environment.
School of PET
- study the needs of laboratory autonomy across multiple highly-interdisciplinary laboratories, and by engaging with existing human-interaction expertise within the ALBERT cohort,
- develop an open hardware platform capable of integrating with a variety of laboratory-based equipment, using existing frameworks as a starting point,
- integrate these devices with both Home Assistant and eLabFTW (an open-source electronic laboratory notebook) and develop meaningful interventions,
- develop guidance and documentation to enable your work to be accessible to non-automation specialist researchers,
- publish and promote your findings and code for both the academic and open-source communities,
- explore the opportunity to commercialise your work, using open-source-centric business models.
Department of Computer Science
Dr Poonam Yadav and Dr Elena Geangu
Research hypothesis: ARL environments are complex systems that require continuous, time-critical communication among autonomous components, such as mobile robots, to perform independent actions. In the best-case scenario, the system is assumed to work as designed. In contrast, in the worst-case scenario, it is assumed that the communication failure of a single component may halt the entire system, compromising efficiency and safety. However, we hypothesise that in real-world environments, ARL systems may partially work, without complete failure. This can affect the judgement and behaviour of lab technicians and assistants who are automating experiments using human-robot interactions. For example, they may choose to continue the experiment or stop the entire operation. Therefore, in this research work, we would like to explore the effects of human behaviour on ARL operations and quantify communication uncertainties in domain-specific (e.g., chemistry) robot-robot and human-robot interactions.
Background context: Robot failures in generic settings have been researched in recent years [1, 3]. However, with the advancement of autonomous systems, more detailed research and understanding of failures and their different impacts (functional, safety, social, psychological, and emotional) in specialised settings and environments is needed. Wireless and wired communication are critical components of distributed robotic systems and play a vital role in the system's resilience and robustness [2]. However, wireless failures have been studied from the technical (functional, security) point of view, and there is still a lack of research on how they impact other robots and humans within the system, especially in domain-specific lab settings. Additionally, the literature does not clearly define the best methodology for measuring all impacts. For example, how long do the impacts last? How long do humans in the lab remain in a more conscious mode after a robot's delayed action or complete stop? Therefore, this is an interesting research direction worth exploring to build smart autonomous lab environments.
Research Objectives
The objectives of this research are to:
- Identify and characterise the different types of communication uncertainties that can occur in ARL environments.
- Investigate the effects of communication uncertainties on the judgement and
behaviour of lab technicians and assistants. - Develop and evaluate strategies for mitigating the negative effects of communication
uncertainties on human behaviour in ARL environments.
Methodology
- A better understanding of the different types of communication uncertainties that can occur in ARL environments.
- A better understanding of the effects of communication uncertainties on the judgement and behaviour of lab technicians and assistants.
- Effective strategies for mitigating the negative effects of communication uncertainties on human behaviour in ARL environments.
Significance
Key references:
[1] Cecilia G. Morales et. al, Interaction Needs and Opportunities for Failing Robots. In Proceedings of the 2019 on Designing Interactive Systems Conference (DIS '19)., https://doi.org/10.1145/3322276.3322345
[2] V. Kumar, P. Yadav and L. S. Indrusiak, "Resilient Edge: Building an adaptive and resilient multi-communication network for IoT Edge using LPWAN and WiFi," in IEEE Transactions on Network and Service Management, doi: 10.1109/TNSM.2022.3228291.
[3] Honig S and Oron-Gilad T (2018) Understanding and Resolving Failures in Human-Robot Interaction: Literature Review and Model Development. Front. Psychol. 9:861. doi: 10.3389/fpsyg.2018.00861
Department of Chemistry
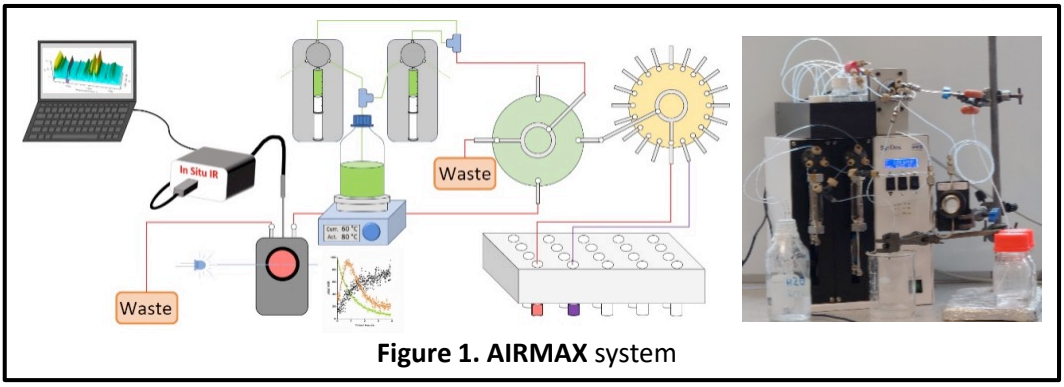
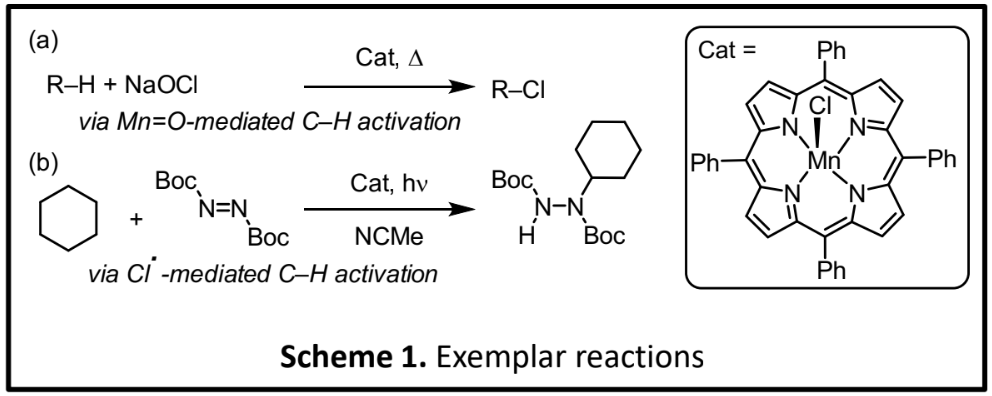
School of PET
Dr. Jihong Zhu (PET), Prof. Andy Tyrrell (PET) and Prof. Ian Fairlamb (Chemistry)
- Identify key manipulation skills in chemistry “Schlenk line” experiments
- Implement learning from demonstration techniques to enable the robot to acquire and refine these skills through observation and imitation.
- Evaluate the performance and effectiveness of the robotic chemist in comparison to traditional human-led experimentation.
- A robotic system capable of autonomously conducting chemistry “Schlenk line” experiments.
- Demonstrated effectiveness of learning from demonstration techniques in enhancing the robot's performance.
- Insights into the advantages and limitations of robotic chemists compared to traditional human-led experimentation - a side-by-side comparison, enabling process verification, reliability and reproducibility to be established.
School of PET
C. Ridgers, C. Arran (School of Physics, Engineering and Technology), S. Wright (Department of Computer Science).
Since the invention of the laser in the 1960s advances in technology have enabled us to reach ever higher laser power. Current lasers operate at the PW level, with 10-100PW laser systems in development. This is interesting because when such high power lasers interact with matter they rapidly ionise the material to create a plasma. The electric and magnetic fields in such a plasma are so strong that they can accelerate particles to GeV energies in millimetres (compared to many metres in a conventional accelerator). As the electrons are accelerated they also radiate copious amounts of energy as hard x-rays. Such compact particle and radiation sources have many transformative applications in imaging requiring high brightness but spectral tuneability (medicine to materials inspection) and very fast (femtosecond) time resolution (imaging inertial confinement fusion experiments). This range of applications has provided motivation for the UK government to fund the £85M Extreme Photonics Applications Centre (EPAC) at the Rutherford Appleton laboratory (RAL) in Oxfordshire.
Since the invention of the laser in the 1960s advances in technology have enabled us to reach ever higher laser power. Current lasers operate at the PW level, with 10-100PW laser systems in development. This is interesting because when such high power lasers interact with matter they rapidly ionise the material to create a plasma. The electric and magnetic fields in such a plasma are so strong that they can accelerate particles to GeV energies in millimetres (compared to many metres in a conventional accelerator). As the electrons are accelerated they also radiate copious amounts of energy as hard x-rays. Such compact particle and radiation sources have many transformative applications in imaging requiring high brightness but spectral tuneability (medicine to materials inspection) and very fast (femtosecond) time resolution (imaging inertial confinement fusion experiments). This range of applications has provided motivation for the UK government to fund the £85M Extreme Photonics Applications Centre (EPAC) at the Rutherford Appleton laboratory (RAL) in Oxfordshire.
In a recent publication of a Gemini experiment we showed that, by incorporating automated robotic control of the laser’s adaptive optic, we were able optimise hard x-rays from a laser-based accelerator – see figure 1. The Bayesian optimisation algorithm we used enabled us to rapidly explore the parameter space but also predicted an optimum we had not expected. An unexpected correlation between the phase components (introduced by the adaptive optic) and the electron beam charge was found. The physical reason for this is still not understood.
Figure 1 – Left: experimental setup for high-power laser particle acceleration. The laser accelerates the electrons in the plasma. The accelerated electrons emit x-rays. The electrons are separated from the x-rays by a magnet and both are measured on a shot-by-shot basis. Right: These measurements are fed to the Bayesian algorithm which determines which regions of parameter space to explore on the next iteration, doing so by robotically controlling the adaptive optic. As the number of iterations increases (n) the uncertainty in the model (in this case for x-ray brighness) is reduced. Taken from our paper Shalloo et al. Nat. Comms. 11 6355 (2020).
This project will address the many outstanding questions in automated control of laser-plasma experiments. For example: Can the automated control loops reveal new nonlinear plasma physics, difficult to predict using our prior ‘human controlled’ methodologies? The unexpected correlation between phase components in our proof of concept experiment suggests so. What do we do when the automated experiments predict unexpected results? The physics underlying the result of our automated Bayesian control is often unclear yet a clear physical understanding not only gives us confidence in the results but also suggests new avenues for investigation (for example other parameters we could robotically control). These questions are at the heart of how we integrate the necessary robotic automation into high power laser research in the most effective way.
This project focusses on two important areas of automation in laser-plasma experiments, critical to EPAC.
- Can we construct robotic automated systems for all the important aspects of a high power laser based accelerator? We have started with the adaptive optic but we will also need to automate the target properties – typically a gas (so pressure, composition). The student will design and build such a system.
- Can we build a research methodology to best incorporate this robotically controlled experiment? Specifically we will investigate how to best understand unexpected results (such as the correlations with phase discussed above). In the Physics and Computer Science Departments we typically work together to use large-scale simulations but we will need to accelerate these to explore the identified parameter regimes. This could be done using modern computational architectures or using surrogate, neural network based models.
This project offers the opportunity to gain hands on experience building robotic control systems for state-of-the-art experiments and to gain proficiency with high performance computing and AI methodologies. The student will also gain experience working in a large team to deliver experiments on the world’s highest power lasers.
School of PET
Dr Manish Chauhan, School of PET, Dr Neil Hunt
Project context:
Ultrafast 2D-IR spectroscopy is a new variant of Infrared spectroscopy which offers a unique analytical method for characterizing complex biomolecular samples. The bonds between atoms in molecular structures absorb IR light at specific wavelengths, so IR spectroscopy provides valuable information about the functional groups, chemical composition, and molecular structure of compounds. 2D-IR spectroscopy measures a two dimensional ‘molecular fingerprint’ which is unique to a given molecule and which has been shown to be extremely sensitive to protein structure. Thus 2D-IR has shown considerable promise as a tool for cancer detection and diagnosis because it is the only method with the potential to measure and quantify diagnostically relevant changes to protein levels in patient blood serum. New laser technology means that a 2D-IR spectrum can be measured in ~ 1 min per sample, which makes sample preparation the rate determining step. Robotic automation of sample preparation, presentation and measurement for protein-rich fluids could pave the way to
- Biomolecular fingerprinting of proteins, lipids, nucleic acids, and carbohydrates, resulting chemical composition of biological fluids. This helps in cancer detection and characterization.
- Liquid biopsy analysis via IR and 2D-IR spectroscopy could provide insights to guide accurate diagnosis of cancer and determination of its stage/grade.
- Monitoring treatment efficacy via infrared spectroscopy of biofluids could help in monitoring effects of cancer treatment (like chemotherapy).
- Preparation and measurement of protein samples in combination with candidate drug molecules could be used to facilitate high throughput screening of the structural impact of drugs binding to proteins, with benefit to the pharmaceutical sector.
- Extending beyond liquids to tissue characterization could allow visualization of tissue sections at microscopic level. This technique can identify cancerous regions with tissue samples.
Hence, infrared, and 2D-IR spectroscopy can offer valuable insights into the characterization of proteinaceous samples, leading to understanding of molecular problems relevant to biomedical applications. Despite its advantages, 2D-IR spectroscopy suffers from limitations like:
- Need for high sample concentrations (millimolar range), which implies preparation for infrared and 2D-IR spectroscopy of water-rich protein-containing fluids is time consuming and requires specialized training.
- As a result of high sampling time, data acquisition for samples is time consuming. Other technical challenges involve phase distortion, vibrational mode coupling, solvent effects, which makes spectrum analysis difficult as it is computationally demanding.
The proposed research aims to integrate 2D-IR spectroscopy with robotic automation for biomolecular fingerprinting of cancer. This could realize projects aiming to establish the link from 2D-map to protein 3D structure, accelerating structural biology research and improving our ability to quantitatively analyze patient biofluid samples. Robotics has the potential to revolutionize bio-photonic identification of cancer with benefits involving increased productivity, accuracy, efficiency, and efficacy. The PhD project will involve interdisciplinary research in:
- Finding robotic solutions for automated biological sample preparation (liquid or tissues) and delivery. These robotic solutions are important for achieving high throughput and limiting effect on potential sample contamination or degradation through handling.
- Developing robotic techniques for collecting high-quality spectral data. This ability to make and measure samples from constituent components (e.g., drugs, proteins) before passing the samples for measurement could lead to fully automated drug-screening protocols.
- Developing Machine learning methods for automating data analysis and overcoming computational limitations. Automation would remove human error in sample preparation, which would enable reliable collection of large datasets that could be compared using ‘big data’ methods such as Machine Learning.
Successful candidate will work with an interdisciplinary team of researchers from Chemistry, Robotics, Physics and Biology department at the University of York. Clinical partners will help in validating results during the research work.
Key areas of research:
- Design, control of robotic automation for spectroscopic sample preparation.
- Developing Machine learning algorithm for analysing spectroscopic data.
- Utilizing AI/ML algorithms by co-relating them with micro-biological information for cancer diagnosis.
- Data analysis using mathematical modelling.
Possible areas of impact
Novel Collaboration for synergizing interdisciplinary research (robotics, optical science, spectroscopy, biology, AI and ML) for cancer diagnosis.
Training provided:
Research will include working with synthetic biochemical compound, cancerous tissues, mechanical and electronic circuit designs, prototyping and pre-clinical in-vitro testing of robotic devices.
- Exploring spectroscopic data and relating it with chemical bonding of biological samples.
- Integration of sensors, camera interfaces into robotic systems.
- 2D-IR spectroscopy training will be provided.
- AI/ML modelling and data analysis using CNN.
Candidates with the following skills are desirable::
Proficiency in Data Analysis/AI/Machine learning (Python, MATLAB), programming (Micro-controller/processors), mechanical CAD design (SolidWorks, hands-on experience of 3D printing, laser cutting, 3D scanning). Candidate should be able to understand chemistry from basic concepts especially fundamentals of spectroscopy.
Department of Computer Science
Dr. Pedro Ribeiro (CS) and Dr. Jihong Zhu (PET)
Introduction
Chemistry plays a major role in addressing society’s needs, such as preventing disease and ensuring food safety. Currently, laboratory experiments require a high degree of human intervention, limiting throughput and presenting safety risks. Increasing the automation of laboratory experimentation tasks has the potential to deliver gains in productivity and safety.
Human-robot collaboration can be beneficial to assist chemists in safely preparing and running chemical reactions. A robot can monitor progression and take action when hazards arise. Post reaction a robot can also assist with handling unknown or potentially harmful substances, for example, via joint human-robot manipulation of vials and use of rotary evaporators to remove reaction solvents. Furthermore, in complex experiments with multiple steps, a collaborative robot could make the process more efficient. The robot can prepare the next step, such as preparing vials, while the human is working on the current step, reducing the overall time taken.
However, demonstrating that robots can work safely and effectively together with humans in a lab setting is currently challenging. For example, what mathematical models are best suited to capture human-robot interactions (HRI), given the variability among humans and the adaptation that naturally occurs as humans get more familiar with a robot? Even if we consider specific scenarios, there is a need to tailor verification techniques to the domain of ALBERT. Existing techniques assume static scenarios and or consider a limited number of assumptions regarding the entities in a scenario and the dynamics of moving agents.
This research project aims to investigate the modelling and verification of human-robot collaboration in the context of laboratory experiments. The aim is to explore the use of formal verification techniques throughout the development of a robotic system to ensure safe and reliable interaction between humans and robots during an experiment.
Objectives
- Characterise laboratory task(s) suitable for human-robot interaction (HRI).
- Model HRI scenarios for a task involving the robot and humans.
- Develop and tailor formal verification techniques to guarantee the safety and correctness of HRI during collaborative experiments.
- Evaluate the performance, efficiency, and safety of the collaborative chemistry experiments compared to traditional human-led approaches.
Methodology
- Drawing on domain-expertise from chemists, characterise laboratory task(s), for example, joint human-robot manipulation of rotary evaporators, where HRI could be beneficial.
- Identify tasks from the literature where human-robot automation could be appropriate.
- Liaise with research and technical staff in Chemistry and conduct interviews/surveys.
- Draw up a list of requirements for the Human-Robot Collaborative System (HRCS)
- Design or adopt a Human-Robot Collaborative System suitable for the task:
- Consider and or design a robotic platform capable of collaborating with human chemists in a shared workspace suitable for meeting the identified requirements.
- Consider and or design perception and planning algorithms to enable the robot to understand and respond to human actions and intentions.
- Ensure seamless communication and coordination between the human and robot for efficient task allocation and execution.
- Formal Verification of HRI:
- Apply formal techniques to model and analyse the interaction between the human and robot in scenarios for human-robot teamwork in chemistry experiments.
- Develop formal specifications that incorporate safety constraints to ensure the correctness of the collaborative system's behaviour.
- Employ and tailor formal verification techniques such as model checking or theorem proving to verify the compliance of the system with the specified properties.
- Experimentation and Evaluation:
- Define a set of chemistry experiments that require close collaboration between the human and robot.
- Conduct experiments using the collaborative system and collect data on performance, efficiency, and safety measures.
- Compare the results with traditional human-led or robot-led experiments to assess the benefits and limitations of the collaborative approach.
Expected Outcomes
- A collaborative robotic system capable of effectively working alongside human chemists in conducting chemistry experiments.
- Tailored formal verification techniques for ensuring the safety and correctness of human-robot interaction during collaborative work.
- Insights into the advantages and challenges of human-robot collaboration in chemistry experiments, along with the benefits of formal verification.
Training offered
The candidate will be able to attend graduate-level modules in the School of Physics Engineering Technology and the Department of Computer Science relevant to the research, such as Machine Vision and Human-Machine Interaction and Assurance & Proof. They will be able to draw on domain-expertise of the Fairlamb Group, led by Prof. Ian Fairlamb, at the Department of Chemistry, facilitated by interaction with Dr. Chris Horbaczewskyj. The university will provide training on a rich set of skills, such as, research management, leadership, academic writing, employability and public speaking. The student will also be able to take advantage of a very successful university-wide mentoring program. The student will benefit from membership of the RoboStar Centre of Excellence in Software Engineering for Robotics and training by the York Graduate Research School.
Facilities and equipment
The candidate will benefit from the facilities of the Institute for Safe Autonomy, home to over 100 experts from multiple disciplines. Specific equipment, such as a Franka Emika robot, of the Robot-Assisted Living LAboratory (RALLA) led by Dr. Jihong Zhu, will be available for the research. See full list here.
References
Othman U, Yang E. Human-Robot Collaborations in Smart Manufacturing Environments: Review and Outlook. Sensors (Basel). 2023 Jun 17;23(12):5663. doi: 10.3390/s23125663. PMID: 37420834; PMCID: PMC10304173.
Kress-Gazit, H. et al., 2021. Formalizing and guaranteeing human-robot interaction. Communications of the ACM, 64(9), pp.78-84.
Foster, S. et al., 2021. Hybrid systems verification with Isabelle/HOL: Simpler syntax, better models, faster proofs. In FM 2021, November 20–26, 2021, Proc. 24 (pp. 367-386). Springer.
Miyazawa, A., Ribeiro, P. et al., 2019. RoboChart: modelling and verification of the functional behaviour of robotic applications. Software & Systems Modeling, 18, pp.3097-3149.
School of PET
Main Supervisor: Dr Mahmoud Dhimish (MD) (Assistant Professor in Electrical Engineering and Head of AI for Sustainable Engineering Lab, School of Physics, Engineering and Technology)
Co-Supervisors: Professor Miles Elsden (ME) (Director of the Institute for Safe Autonomy, Institute for Safe Autonomy), Dr Richard Douthwaite (RD) (Reader in Chemistry, Department of Chemistry)
Introduction & Background. Solar photovoltaics (PV) are a cornerstone in the global shift towards renewable energy, contributing close to 4% of worldwide electricity generation. The key to enhancing solar PV efficiency lies in the advanced chemical synthesis of materials, a process crucial for improving their ability to absorb and convert sunlight into electricity. This project focuses on developing new semiconducting materials and nanostructures through chemical synthesis, aiming to optimise their light-harvesting capabilities. Such advancements are vital for enhancing the efficiency, stability, and cost-effectiveness of solar cells. Therefore, when discussing chemical synthesis in the context of solar panels, the emphasis is on the fabrication and composition of the semiconductor materials and components utilised in panel construction, rather than the photophysical processes responsible for the conversion of light into electricity within the panels.
Present challenges in Solar PV material synthesis include reproducibility of processes (e.g., solvothermal reactions1 and solid-state reactions2 ) and their impacts on PV cell performance. Automation, supported by the precision and adaptability of robotics and AI, offers a transformative solution. Robots can execute tasks with high accuracy, while AI facilitates real-time decision-making and understanding of complex chemical processes. This integrated approach promises a significant leap in solar PV material synthesis, aligning efficiency with sustainability. By coupling robotics and AI, we stand at the precipice of a transformative approach to Solar PV material synthesis—an approach that marries the unerring accuracy of robots with the nuanced intelligence of AI algorithms3.
PhD Project Aim. This PhD project endeavours to harness the synergy of robotics, AI, and advanced chemical processes for pioneering solar PV material synthesis. It aims not only to set new benchmarks in efficiency, safety, and sustainability but also to innovate in the quality control and testing of newly developed solar cells. A key addition to this project will be the utilisation of Electro-photoluminescence (EL/PL) imaging techniques that will be employed to rigorously test the newly synthesised solar cells, providing critical insights into their efficiency and defect profiles. This comprehensive approach ensures a holistic development process - from material synthesis to final quality assurance. The key Objectives of this project are:
- Robotic System Design and Prototyping for New Solar Panel Material Synthesis. Develop and prototype a robotic system using CAD and simulation tools, tailored for the chemical synthesis of innovative solar panel materials. The system will be designed to create an environment for synthesising new compounds and structures to boost solar cell efficiency. The prototype will undergo iterative testing and refinement, ensuring support for the synthesis process with high quality and consistency standards. This phase will also include the integration of EL/PL imaging systems for quality testing of the new solar cells.
- Integration of Sensory Technologies for Enhanced Chemical Synthesis in Solar PV. Enhance the chemical synthesis process by integrating advanced sensors. These sensors will identify and monitor the properties of compounds used in solar PV synthesis and oversee the chemical processes to optimise light-harvesting capabilities. They will ensure the consistency and effectiveness of material development. Environmental monitoring sensors will also be deployed to maintain a stable and controlled synthesis environment, crucial for the efficient conversion of solar energy.
- Real-time AI-Driven Decision Making. Data Processing: develop algorithms to expeditiously analyse data from the embedded sensors.Adaptive Decision-Making: infuse AI capabilities that enable the robotic system to amend its operations based on instantaneous feedback, preserving the highest synthesis standards.
- Safety, Ethical Considerations, and Process Optimisation. Safety Algorithms: Implant AI algorithms predicting potential hazards, prompting the robot to pre-emptively act. Ethical Framework: Develop a regulatory and ethical blueprint for the autonomous synthesis process, emphasising human-robot collaboration, data privacy, and environmental impact. Process Efficiency: Scrutinise the entire synthesis protocol, spotlighting and rectifying inefficiencies, achieving a harmonious blend of swiftness and quality.
Fit & Added Value to CDT. This project integration of robotics and AI into Solar PV synthesis epitomises the ALBERT CDT's mission to revolutionise laboratory automation, fostering cleaner, more efficient processes and safer lab environments. This initiative directly addresses key ALBERT queries, such as enhancing human-robot collaboration and reducing dependence on specialised equipment. By automating chemical synthesis, we extend the capabilities of robots within the lab, simultaneously addressing technological advancements and the sociotechnical dynamics of such integration—core to ALBERT CDT's multidisciplinary approach. This PhD project will benefit from a rich network of experts, fostering a collaborative milieu that is in sync with our aims in the Solar PV field. The CDT's commitment to reshaping laboratory practices through autonomous robotics merges with our project goals, promising innovative breakthroughs. The continual learning environment afforded by the CDT's workshops ensures our methodologies stay at the forefront of scientific innovation. Thus, ALBERT CDT's resources and expertise are instrumental in amplifying the project's scope and impact, setting the stage for transformative research outcomes.
Contribution to CDT. Dr MD will develop skill hands-on training on "Real-Time LabVIEW Software Simulation," as a full-day session. He will also deliver two intensive workshops, each lasting three to four hours, focused on "Engineered Intelligence: The Convergence of AI and Robotic Systems" and "Navigating the Data Revolution in Chemistry". Prof ME will design and deliver a half-day training session on "Next Generation Safety Autonomy," which promises to delve into the advanced facets of autonomous systems and their safety implications. Dr RD delivers ten one-hour lectures in the "Chemistry of Inorganic Materials and Nanoparticles" that incorporates many of the materials, synthetic processes, and photochemical properties relevant to solar cell fabrication and function. These educational sessions will be made available to the entire cohort of the ALBERT CDT programme, benefiting both existing members and incoming students.
Available Resources. The PhD project is based in the AI for Sustainable Engineering Lab at the School of Physics, Engineering, and Technology. The student will have access to advanced lab tools, such as the Atomic Layer Deposition (ALD) system, for solar cell development and PV material analysis. A key resource is the Institute of Safe Autonomy's new 200kW PV array system, a £800K investment featuring robotic data monitoring. This system is crucial for the student's research testing and development. Additionally, the chemistry department offers extensive facilities for material synthesis and analysis, including composition, structure, and photophysical properties, supported also by the York JEOL Nanocenter resources.
Industrial Collaboration. Above Surveying Ltd, the UK's leading solar PV digitisation company based in Colchester, will provide a valuable industry experience for our PhD student. They will host the student for 6 months, offering practical application and enhancement of their solutions, focusing on objectives 1 and 2. Additionally, we have an exclusive partnership with AI giant Google DeepMind in London. The PhD candidate will have access to DeepMind's advanced real-time AI platform, aiding in achieving objectives 3 and 4. DeepMind will also offer the student up to six 2-hour consultation sessions annually and specialized training on their deep learning models, ensuring the student's expertise in AI development and contributing to the project's success.
Supervisory Team. Dr. MD, a top solar PV expert with over 80 journal publications, was named one of the UK's top 5 scientists in 2022. He has secured significant funding, including the £390K EU958223 project, and leads research at the PV farm in the Institute of Safe Autonomy. He has successfully mentored three PhD students. Professor ME, with a PhD in applied mathematics, has a varied background in academia, industry, and government. As the director of the Institute of Safe Autonomy and the PI of the £1.52M UKRPIF net-zero project, he formerly served as Chief Scientist at the Department for Transport, focusing on AI-Robotics challenges. Dr RD has expertise in chemical materials synthesis from molecules, to condensed solids and their composites for photophysical and photochemical applications used in PVs. This has included extensive funded (EPSRC/industry) collaborations with colleagues across physics and engineering.
- Yao, et al., ACS Applied Materials & Interface. 14, 7493-7503 (2022).
- Rahman et al., Polymer. 230, 124092 (2021).
- Winfield, A., Nature Electronics. 2, 46-48 (2019).
School of PET
Dr. Babar Jamil and Dr. Pedro Ribeiro
Introduction
Society relies on Chemistry processes to fulfil basic needs, such as ensuring water and food are safe for consumption. Laboratorial experiments often involve several manual processes, which is not only a bottleneck but also puts humans unnecessarily at risk from manipulating harmful chemicals. In this context, the introduction of robotic systems has the potential for increasing safety and productivity. However, the variety of assets that must be handled by a robotic system in a laboratory presents challenges for their safe and efficient manipulation.
Project Objectives and Aims
This project aims to develop a novel robotic system capable of extending and handling/manipulating Microtiter Plates (made of metal) with 48 vials and liquid chemicals, for example, as used in a robotic sample preparation system, that can weigh up to 1.5Kg and which are often required to be moved from one machine to another for experiments by the chemists. This often is a challenging task that needs chemists to pick and place these plates which can be automated using extensible robotics. The aim is to make an autonomous mobile robot with extensible robotic arm that can work in ALBERT to carry out these repetitive and sensitive tasks while also ensuring the safety of machines and humans. The inherent compliance of this robot will allow it to be safer around objects and humans.
One of the main questions or challenges this project will address is whether it is possible to develop a robotic arm that can extend and enable a robotic arm to move through unstructured environments while also ensuring a load-bearing capacity to interact with objects. To achieve this, we will investigate the development and integration of a novel extensible robotic arm capable of extending over 400% of its initial length and carrying a payload of over 2 kg. This capacity will ensure that the robot is able to handle both the Microtiter Plates, the plates handling gripper/tool, and any future modifications necessary for conducting experiments.
A crucial aspect will be the development of a cyber-physical model of the robot that enables simulation in a virtual environment, interacting with Virtual Microtiter Plates prior to actual deployment in the lab. To this end, modern diagrammatic model-based approaches will be exploited that enable the automatic generation of artifacts for simulation. Such approaches will be tailored for the task at hand and any lessons learned from their application will be used to drive further research in that area. This will require developing mathematical models for the robots and subsequent testing on the actual robotic platform for verification.
To chieve these goals, Ph.D. students will work on designing improvements for the available design/system, completing mathematical modelling under supervision, verifying mathematical models through experimentation, and then developing and integrating simulation software to model these robots through software. Finally, a novel extensible robotic manipulator will be developed and tested in an experimental setting.
Requirements and Challenges
Following are the challenges of this project:
- Well equipped with programming skills, most importantly object-oriented programming, comfortable with programming languages including C/C++, Python, ROS.
- Understanding of mathematical modelling of dynamical systems and control theory.
- Design and implementation of an extensible robotic platform which can extend as well as to carry pick and place tasks to a payload (Microtiter Plate and gripping tool) of 2Kg. To solve this, we will do rigorous design and simulation testing with some of the available software. This will require the student to be well equipped with mechanical design software (Inventor + COMSOL/ANSYS).
- The project will require hands-on experience with electronics such as PCB design, circuit design etc.
Training
The candidate will be able to attend graduate-level modules in the School of Physics Engineering and Technology and the Department of Computer Science, as relevant to their research. They will also be able to draw on domain-expertise from the Fairlamb group at the Department of Chemistry, facilitated by interaction with Dr. Chris Horbaczewskyj.
The university also provides general training on a diverse range of skills, such as, research management, leadership, academic writing and public speaking. The candidate will also benefit from a successful university-wide mentoring programme.
The student will benefit from membership of the RoboStar Centre of Excellence in Software Engineering for Robotics, and training offered by the York Graduate Research School.
Facilities and equipment
This research will mostly be carried out at The University of York and Institute of Safe Autonomy (ISA). ISA has been built for state of the art research in safe autonomy and robotics. The labs are equipped with resources required for this project such as, 3D printers, electronics lab, manufacturing lab, computational resources, mobile robots, manipulators, vision systems, motion trackers, etc. We are also seeking additional funding to secure equipment to further enhance the availability of resources.
Literature
- Babar Jamil, Gyeongjae Yoo, Youngjin Choi and Hugo Rodrigue, “Hybrid Robotic Manipulator using Sensorized Articulated Segment Joints with Soft Inflatable Rubber Bellows”, IEEE Transactions on Industrial Electronics, vol. 69, No. 10, pp. 10259-10269, Oct., 2022.
- Keyvanara, M.; Goshtasbi, A.; Kuling, I.A. A Geometric Approach towards Inverse Kinematics of Soft Extensible Pneumatic Actuators Intended for Trajectory Tracking. Sensors 2023, 23, 6882.
- X. Chen, Y. Guo, D. Duanmu, J. Zhou, W. Zhang and Z. Wang, "Design and Modeling of an Extensible Soft Robotic Arm," in IEEE Robotics and Automation Letters, vol. 4, no. 4, pp. 4208-4215, Oct. 2019
- Chen, X., Zhang, X., Huang, Y., Cao, L., & Liu, J. (2022). A review of soft manipulator research, applications, and opportunities. Journal of Field Robotics, 39, 281–311.
- A. Miyazawa, A. Cavalcanti, S. Ahmadi, M. Post, J. Timmis: RoboSim Physical Modelling Reference Manual. University of York (2020). Available online: https://robostar.cs.york.ac.uk/publications/techreports/reports/physmod-reference.pdf
Department of Computer Science
Dr. Xinwei Fang (CS), Dr. Jihong Zhu (PET)
Introduction:
Recent advances in AI and robotics have enabled robots to perform highly precise tasks in settings `such as chemistry laboratory experiments (Arthur, 2020). However, despite stringent quality assurance and the use of advanced sensors, there remains a notable gap in detecting subtle but significant changes in robots (Ng et al., 2022). For instance, the unnoticed gradual wear of a robot's arm joint might result in imprecise solution mixing, thereby compromising the experimental results. This issue acts as one of the major barriers in the deployment of high-autonomy robotic systems within chemical laboratories, a concern shared in the scope of the ALBERT CDT.
Problem Statement:
Many components in a robotic system—especially the physical ones—undergo gradual degradation that often goes unmonitored, limited by the scope of sensor deployment (which is constrained by cost-effectiveness and feasibility). These subtle changes may not immediately affect a system’s primary functions, but they can accumulate and create long-term issues. For instance, dual-armed robotic systems used for transferring and mixing chemical solutions may experience gradual deterioration, leading to a loss of synchronisation and compromised task precision. This can result in poorly mixed solutions and incorrect experimental results. Humans, being naturally good at situational awareness, might detect these issues through subtle indicators like solution colour or clarity. The challenge is to develop robots with comparable situational awareness capabilities, enabling them to be highly autonomous and perform prolonged tasks without human intervention in chemistry laboratory experiments.
Objective and Research Questions:
This PhD project aims to develop a novel framework enabling robots to detect and adapt to operational changes that are not directly monitored by the on-board sensors. The core of this framework will be a stochastic model designed to continuously monitor, analyse, and adapt to the robot's behaviour, pinpointing deviations in operational performance that might otherwise go unnoticed and before they cause issues. This framework will be particularly focused on armed robotic systems used in chemistry laboratories (Jiang et al., 2023). To achieve these goals, the following research questions will guide the project:
- How can robots effectively detect, model, and quantify operational changes that are not directly monitored, utilising available data sources such as sensor readings and system logs?
- What methodologies can be developed and employed to accurately assess the impact of these changes on the overall performance of robotic systems?
- How effective is the framework in identifying and responding to critical changes, and what are the implications in terms of computational needs and resource overhead?
This project contributes to advancing robotic reliability and marks a step forward in future development of high-autonomy robotic systems in assisting with tedious and potentially hazardous chemistry laboratory experiments, which directly connects to the aims of the ALBERT CDT.
Plan of Work:
The selected candidate is expected to undertake the following tasks:
- Conduct a thorough review of existing situational awareness technologies within the robotics field, with a specific focus on applications within chemistry laboratory experiments and the use of stochastic models. This will involve an in-depth exploration of contemporary approaches and their respective benefits and limitations.
- Collaborate closely with experts and stakeholders connected to this CDT. Activities may include conducting interviews or surveys with domain experts, participating in relevant workshops, and attending CDT-organised events or relevant graduate-level modules in the Department of Computer Science, School of Physics, Engineering and Technology, and the Department of Chemistry.
- Design and implement the proposed stochastic modelling framework. Initial development will take place in a simulated environment to allow for rapid iteration and refinement. Insights from the literature review and stakeholder engagement will play a crucial role in guiding this process. Following this, the framework will be applied to and tested on an actual armed robotic system.
- Conduct a series of controlled experiments to rigorously test and evaluate the framework. This will involve assessing its effectiveness in detecting and responding to operational changes, with a focus on the research questions outlined. Collaboration with domain experts will be essential during this phase to ensure that the framework's performance is thoroughly vetted against real-world standards and requirements.
References
Arthur, Amy. 2020. “Meet KUKA, the robot that's tackling chemistry's biggest challenges.” BBC Science Focus, October 20, 2020. https://www.sciencefocus.com/future-technology/kuka-mobile-robot.
Fleischer, Heidl, Robert R. Drews, Jessica Janson, Bharath Patlolla, Xianghua Chu, Michael Klos, and Kerstin Thurow. 2016. “Application of a Dual-Arm Robot in Complex Sample Preparation and Measurement Processes.” SLAS Technology 21, no. 5 (October): 671-681. https://doi.org/10.1177/2211068216637352.
Jiang, Ying, Hatem Fakhruldeen, Gabriella Pizzuto, Louis Longley, Ai He, Tianwei Dai, Rob Clowes, Nicola Rankin, and Andrew Cooper. n.d. “Autonomous biomimetic solid dispensing using a dual-arm robotic manipulator.” Digital Discovery.
Ng, Y. J., Matthew S K. Yeo, Q. B. Ng, Michael Budig, M. A. Viraj, Viraj Muthugala, Bhagya Samarakoon, and R. E. Mohan. 2022. “Application of an adapted FMEA framework for robot-inclusivity of built environments.” scientific reports. doi.org/10.1038/s41598-022-06902-4
Department of Computer Science
Dr John Oyekan (Computer Science), Ian Fairlamb (Chemistry), and Helen Sneddon (Chemistry)
Challenge: Chemistry, as a science, has undergone a transformation in recent decades, driven by the exponential growth of knowledge, increased demand for novel chemical compounds, and a greater focus on sustainability.
Furthermore, chemists are often exposed to hazardous chemicals during the investigation, exploring and synthesis of new materials. In order to advance the creation of new materials, we need to change the paradigm of synthesizing them. The ability of robots and automation to carry out tasks that are dangerous and dull provide a mechanism to exploit for the exploration of novel materials that have not been discovered yet.
Within this evolving landscape of discovering new materials, the quest for understanding reaction complexity and harnessing automated synthesis to create novel chemicals has taken centre stage. Chemical reactions have become increasingly complex, demanding a deeper understanding of diverse reactants, kinetics, and reaction pathways. Multistep reactions, intricate intermediates, and non-linear kinetics are just a few of the complexities that researchers face [1]. Understanding and optimizing these multifaceted processes is a bottleneck in fields ranging from pharmaceuticals to materials science.
However, the traditional laboratory setup, while invaluable, often faces limitations when dealing with the intricacies of modern chemistry. This is where the concept of flexible robotic labs comes into play, offering an innovative solution to the challenges of reaction complexity and automated synthesis. Automation has undoubtedly advanced our ability to tackle complex reactions. Automated systems offer precision, high throughput, and consistent execution. However, the conventional automation systems often lack the adaptability and decision-making capabilities required to manage the diverse spectrum of complex chemical processes. Reacting to changing conditions and unexpected variations is a challenge [2].
Proposal: Flexible robotic labs, a novel paradigm in chemical research, represent a promising solution. These labs integrate advanced robotics, artificial intelligence, and adaptive sensors within a modular and adaptable workspace. Researchers can reconfigure the lab's layout and instrumentation to suit the specific requirements of their projects, thus providing an environment that is both versatile and customizable [3].
This 3 year PhD programme will investigate the following hypotheses: Flexible customization and scalability of Automation Systems Enable Broad Applicability Across Chemical Domains leading to more sustainable chemical processes.
Objectives: The following objectives will be investigated:
Objective 1. Reaction Monitoring and Optimization: One of the primary challenges in automated synthesis is the real-time monitoring and optimization of chemical reactions. Automation systems must be capable of accurately assessing reaction progress, adjusting reaction parameters as needed, and ensuring the desired outcome. Developing robust sensors and control algorithms for various reaction types is a research area that would be investigated through the use of off the shelf components as well as 3D printed parts. This approach would support us in developing automation hardware that is versatile and adaptable to handle different reactors, reagents, and conditions for various reactions
Objective 2. Handling Complexity: Many chemical reactions are highly complex, involving multiple steps, reagents, and conditions. Automating such intricate processes demands advanced programming and robotics capabilities to handle the diverse requirements of different reactions. Research will be carried out to design versatile automation systems that can adapt to various levels of reaction complexity.
Figure 1. The breakdown of tasks and duration of each
Objective 3. Integration with AI and Machine Learning: The integration of artificial intelligence (AI) and machine learning presents a significant opportunity to improve the efficiency of automated synthesis. However, challenges include developing AI algorithms that can accurately predict reaction outcomes, optimize conditions, and adapt to unforeseen variations. This stage of the PhD research will focus on creating AI models capable of handling the complexity of chemical systems. The first step would making use of data from various chemical reaction databases. Also, an investigation will be carried out into the use of Generative AI and Large Language Models to support the discovery of novel chemicals.
Objective 4. Synthesizing a flexible automated framework: Using the results obtained from objective 1 to 3 above, a framework would be developed. This framework would support the development of various flexible automated platforms for other biotechnology and chemistry domains looking to apply both AI and automation in the control of various chemical/biological reactors.
PhD programme tasks (See Figure 1): The objectives mentioned above will be achieved through the following tasks:
Literature review and down selection of drug candidates (6 months): This task would involve an extensive literature review that would look into various chemical and medicinal journals to find a range of potential chemical candidates that are disparate enough but also within the constraints of the physical lab equipment. The idea is that the down selected candidates would be capable of testing the flexibility of the automated framework being developed.
Development of a Virtual Flexible Automation Lab (9 months): This will involve searching for potential software that can be applied in developing digital copies of physical chemistry equipment. The chosen tool must be able to be flexibly reconfigured with APIs to chosen various components from a library and tune them. Tuning the library of components would ensure that any physical constraints are respected.
Development of AI and Optimisation Algorithms (9 months): This task will involve the development of and application of population based many-objectives algorithms. The challenge here would be integrating the constraints obtained from virtual flexible automation lab into the population based algorithms. Another challenge is converting the multiple objectives in the chemical space into a form that the optimisation algorithms would work upon. This work will build upon many-objective algorithm development that is being carried out in Computer Science.
Development and Integration with Physical Equipment (12 months): This task would involve understanding the physical equipment in the laboratory and investigating ways by which they can be adapted to work with the developed flexible automation framework. This work would also involve integrating PC controlled hardware (e.g labview cards) towards controlling various hardware in the lab.
Summary: As technology continues to advance, flexible automation is poised to become a paradigm shifting tool in supporting the investigation of complex reactions and contributing to the advancement of chemical science. The result of this PhD would be a framework as well as associated algorithms that would support the research community in exploring new materials. This would empower researchers to tackle intricate chemical challenges, drive advancements in various industries, and contribute to the sustainable and efficient practice of chemistry.
References:
[1] Hu, Y.J., Chen, J., Wang, Y.Q., Zhu, N., Fang, Z., Xu, J.H. and Guo, K., 2022. Biocatalysts used for multi-step reactions in continuous flow. Chemical Engineering Journal, 437, p.135400.
[2] Melin, J. and Quake, S.R., 2007. Microfluidic large-scale integration: the evolution of design rules for biological automation. Annu. Rev. Biophys. Biomol. Struct., 36, pp.213-231.
[3] Roughley, S.D. and Jordan, A.M., 2011. The medicinal chemist’s toolbox: an analysis of reactions used in the pursuit of drug candidates. Journal of medicinal chemistry, 54(10), pp.3451-3479.
Department of Computer Science
Professors Radu Calinescu and Ana Cavalcanti (Computer Science)
Autonomous and AI systems are an increasing part of daily life. As the range and impact of such systems increase due to fast advances in their underlying technology, UKRI has funded a number of investigatory research “nodes” to address the trustworthiness of autonomous systems.
The research node led by the University of York focuses on the resilience of such systems. An important aspect of resilience is to provide assurance that AI and autonomous systems can and will achieve their goals despite the uncertainty and disruption encountered in real-world environments.
In this exciting PhD studentship opportunity, we are teaming with the University of York’s Centre for Doctoral Training in the area of Autonomous Robotic Systems for Laboratory Experiments (Albert CDT), to explore how AI-enabled autonomous robots can collaborate with humans in carrying out experiments in a Chemistry lab. The robot will need to share space and equipment with humans. It can work side-by-side with humans or continue the work out of hours. The challenges come from the need for awareness of human presence, and from the uncertainty in the environment of the experiments. Humans may change the position of equipment and materials, or may forget to follow some critical procedures, and yet the robot must be able to carry out their tasks resiliently and safely.
The project will investigate neuro-symbolic AI methods to perceive, represent and reason about interactions between lab technicians and researchers and AI-enabled autonomous systems, with the aim to enable their resilient collaboration through coordination and adaptation.
The PhD student will have the unique opportunity to collaborate with a multidisciplinary team from Computer Science and Chemistry, and with stakeholders ranging from lab technicians to roboticists. The studentship is designed to offer exceptional access to resources, training and career development and networking opportunities.
Candidates are invited to familiarise themselves with the aims of the Resilience node and the Albert CDT
The successful candidate will conduct their research under the supervision of Professors Radu Calinescu and Ana Cavalcanti at the University of York.
Apply for this Studentship:
We are seeking a highly motivated candidate who should have, or expects to be awarded, a first-class or 2.1 degree or a master’s degree in Computer Science or any other relevant discipline. Preferred skills include writing, communication, presentation and organization skills. The studentship is open to students holding Home fee status.
To be considered, you must apply online to a full-time on campus PhD in Computer Science at the University of York. and quote the title of this project - Human-AI Collaboration for Resilient Emergency Care - in your application.
Please also include the following in your application:
- A short research proposal (<1,500 words).
- A statement of purpose explaining how your competencies and previous experiences makes you an appropriate candidate for this position.