AAIP launches new guidance to assure the safety of machine learned components
Posted on Thursday 4 February 2021
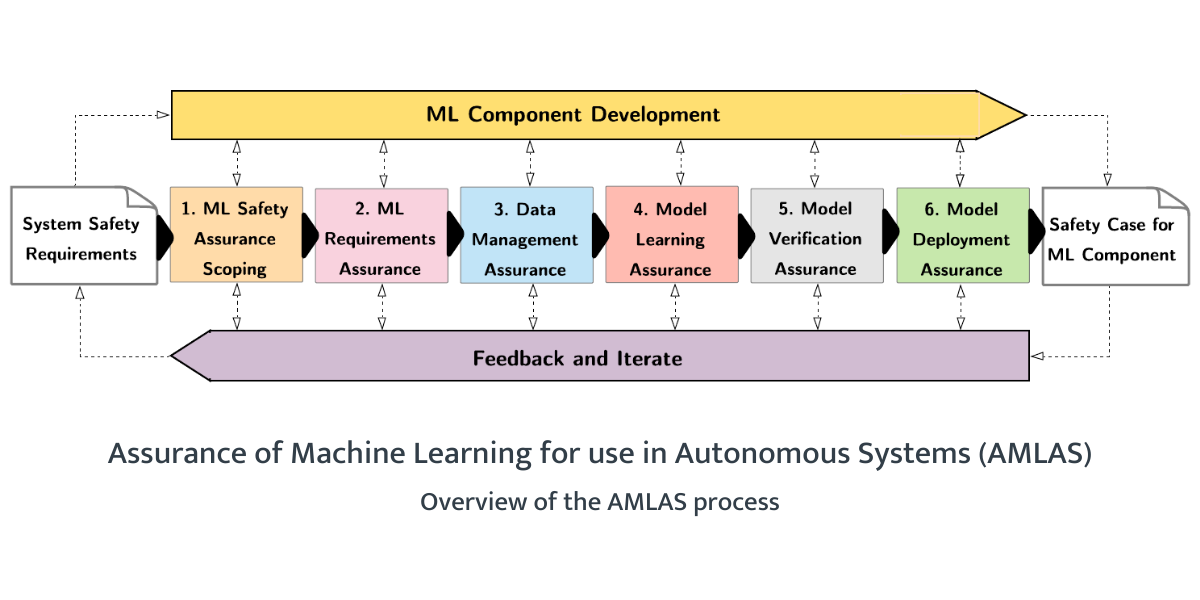
Machine learning (ML) is used in a range of systems that exhibit high degrees of autonomy and are safety-critical. Researchers on the Assuring Autonomy International Programme (AAIP) at the University of York caution that you can’t assure the safety of your system without explicitly and systematically establishing justified confidence in the ML. They have developed a new methodology for assuring the safety of ML components: Assurance of Machine Learning for use in Autonomous Systems (AMLAS).
“We are pleased to publish our AMLAS guidance for safety engineers and developers who are producing ML components to use in autonomous systems,” said Dr Richard Hawkins, AAIP Senior Research Fellow and one of the authors of the guidance.
“We see remarkable developments in ML all the time and many of these components will be used in safety-critical applications in domains such as healthcare or automotive. What we haven’t found is a clear and detailed assurance process that complements the ML development process and that generates the evidence needed to justify the safety of ML components.”
“Without a compelling argument about your ML model to feed into a system safety case, you can’t assure the safety of your system,” continued Dr Habli, Senior Lecturer at the University of York and another of the authors. “AMLAS incorporates a set of safety case patterns and a process for systematically integrating safety assurance into the development of ML components. You can use the patterns provided, follow the process and instantiate the argument.”
The AMLAS process was first presented by Dr Colin Paterson, an AAIP Research Associate, at the SAFE AI workshop in New York in February 2020. Since then it has been reviewed by a wide range of experts from across industry and academia. In the coming months, the process will be evaluated by applying it to a number of case studies in different domains.
Download AMLAS