Assuring the machine learning lifecycle: desiderata, methods, and challenges
The current unprecedented interest in machine learning is fuelled by a vision of its applicability extending to healthcare, transportation, defence and other domains of great societal importance. Achieving this vision requires the use of machine learning in safety-critical applications that demand levels of assurance beyond those needed for current applications.
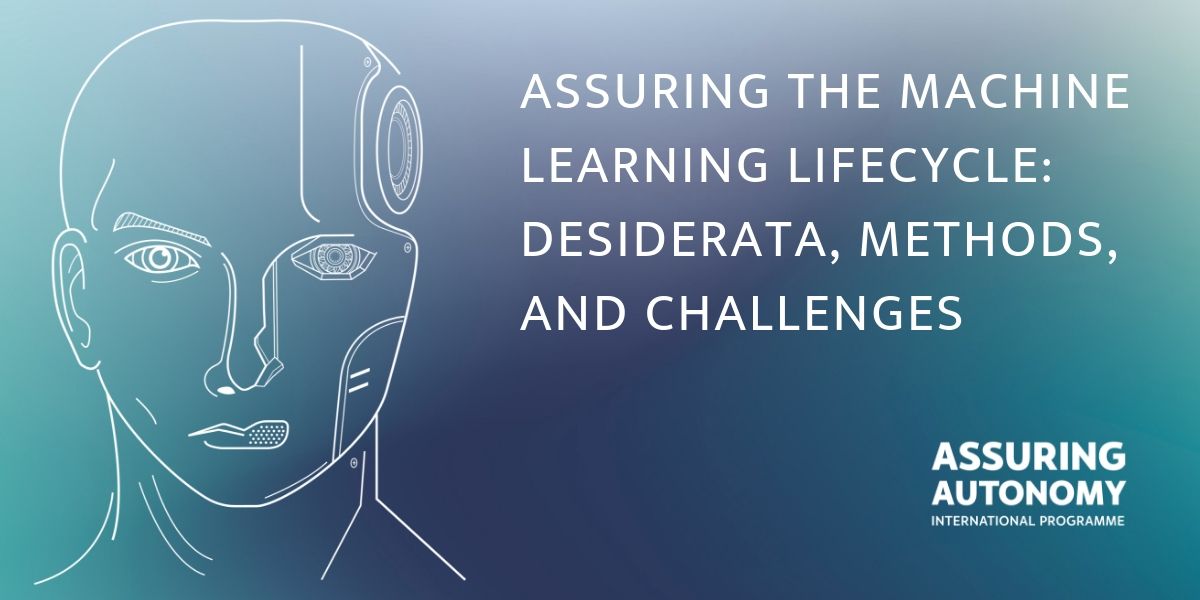
Machine learning has evolved into an enabling technology for a wide range of highly successful applications. The potential for this success to continue and accelerate has placed machine learning at the top of research, economic and political agendas.
This new paper, by Rob Ashmore (AAIP Programme Fellow) and AAIP's Dr Radu Calinescu and Dr Colin Paterson, provides a comprehensive survey of the state-of-the-art in the assurance of machine learning. The survey covers the methods capable of providing the required evidence at different stages of the machine learning lifecycle. The paper begins with a systematic presentation of the machine learning lifecycle and its stages, and then defines assurance desiderata for each stage, reviews existing methods that contribute to achieving these desiderata, and identifies open challenges that require further research.
The paper is under review for journal publication and comments and feedback are welcome. Please email assuring-autonomy@york.ac.uk with your feedback.