Research and practice in arts and creative technologies
Creating a collaborative research and practice community
The School of Arts and Creative Technologies generates new knowledge through scholarly and practice research, through creative and professional practice and through technical innovation.
We pursue a key strategic aim of the University of York, as a university for public good, by engaging in curiosity-driven and action-oriented research. Our work reaches beyond the academy, striving for positive impact through direct outreach to the public and through knowledge exchange with our many partner organisations, including artistic, governmental, non-profit and industrial partners, who in turn help drive our research questions.
 - institutional message.jpg)
The Research Excellence Framework 2021
- All evaluations of our research environment - the support we give our researchers - ranked it as ‘world leading’ or ‘internationally excellent’. 100% of our impact case studies were rated either 'world leading' or 'internationally excellent'.
![]()
The School aims to be a leader, nationally and internationally, in research, scholarship, professional practice and external engagement in the fields of arts and creative technologies. We encourage new ways of thinking about music, theatre, film and television, creative technologies and the creative industries. Through our research and practice we respond to grand challenges by engaging with communities, businesses, governments and cultural organisations.
Dr Lisa Peschel - Senior Lecturer in Theatre and Chair of Research and Practice
Research clusters
The strength and breadth of our research and practice is reflected in five research clusters that help us support our research community and the University's vision for public good.
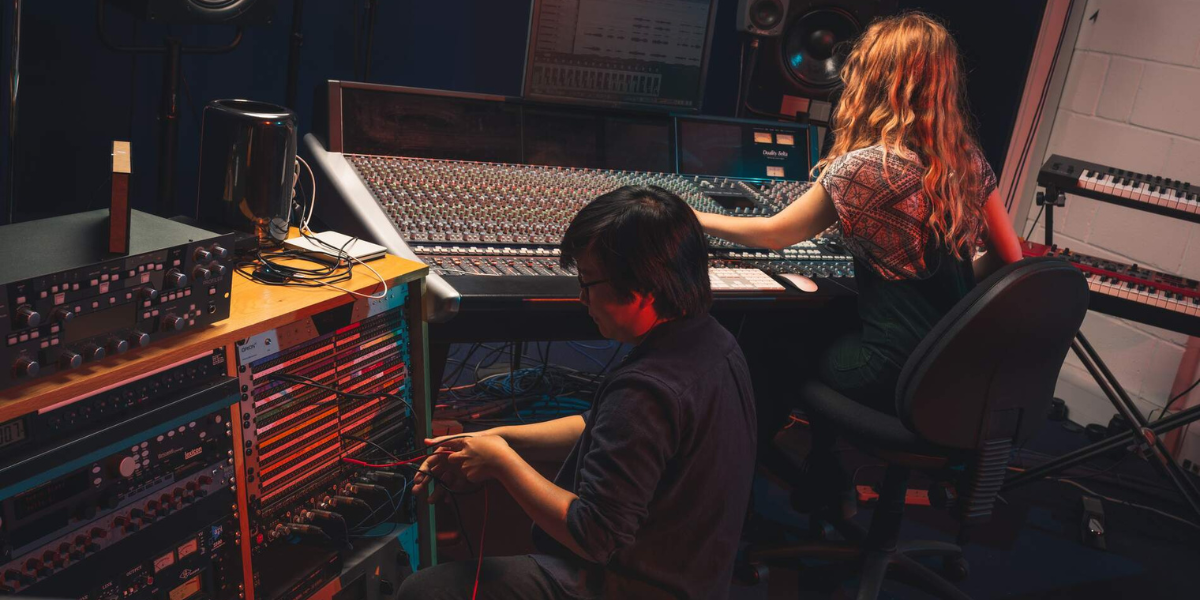
Impact and engagement
We work in innovative ways to further knowledge, make meaningful contributions to culture and society and disseminate our findings broadly and effectively.
Highlights
Facilities
We have a range of facilities to support our research, practice and collaborations with partners.
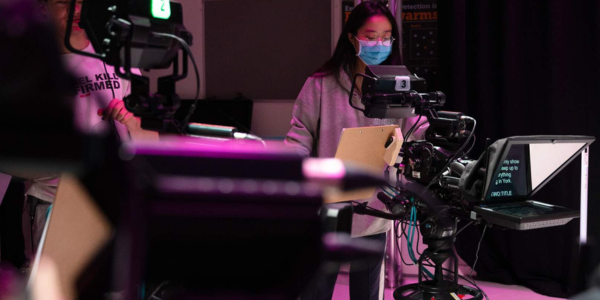
Music press
Research seminar series
To showcase our research, and enhance collaboration, we hold a regular series of seminars including papers by visiting speakers, as well as presentations by University of York staff and current research students.
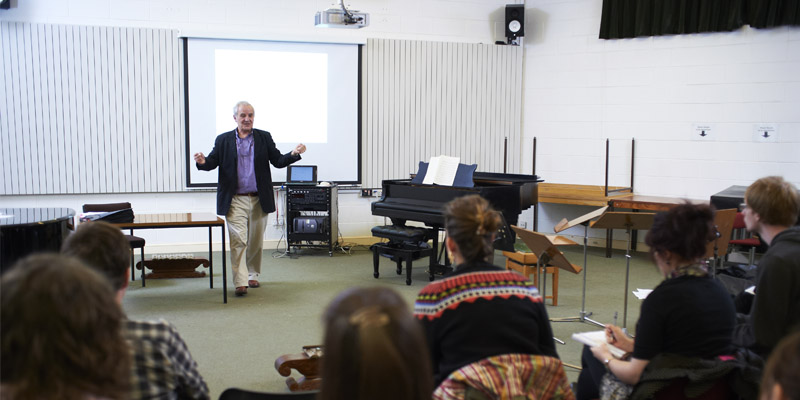
Archives
The Borthwick Institute for Archives houses fascinating collections to support theatre, music and television research and study.