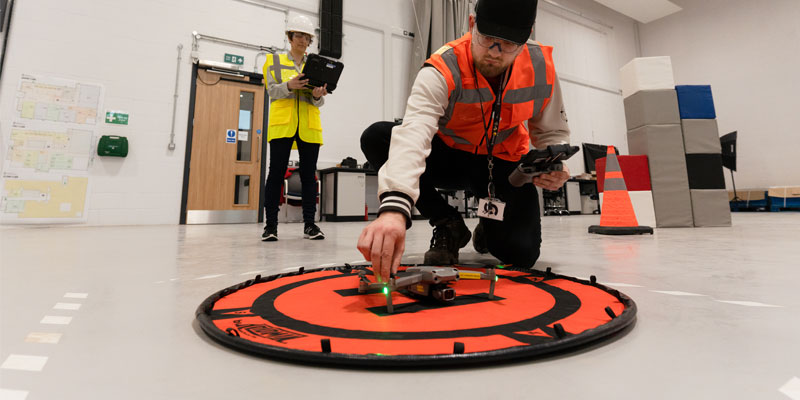
Assurance
We are establishing what is required to demonstrate that any RCAS is safe, and developing robust methodologies to meet these requirements so that the deployment of all such systems is subject to reliable appraisal.
To be able to demonstrate that an RCAS is safe, we need a method that can itself be trusted to be rigorous, transparent and universally applicable.
One of the key barriers to this is that RCAS are making increased use of AI and Machine Learning to adjust themselves to their environment. This means that an RCAS could be demonstrated to be safe one day, but become unsafe the next, which in turn means that we need to design an equally dynamic means of appraising and assuring their safety.
To overcome this barrier, we need to understand the ways in which the use of AI and Machine Learning could lead to the failure of a system; create a system of safety assessments capable of monitoring and interpreting data from RCAS while they are in operation; and build in the potential to update those safety assessments to keep pace with the dynamic evolution of RCAS technology.
These are the challenges addressed by the work of our Assurance research pillar.
![]()
Nobody cares as much about safety as we do at the ISA. The building, the facilities, the disciplinary breadth – it’s all in the service of ensuring and demonstrating that RCAS will not cause harm.
Professor John McDermid, Research Lead.
Activities and Partnerships
Trustworthy Autonomous Systems (TAS)
We manage the Resilience Node of UKRI’s Trustworthy Autonomous Systems Hub, identifying and creating the tools and methodologies required by developers to design RCAS that we can trust to operate effectively within uncertain, changing and disruptive circumstances.