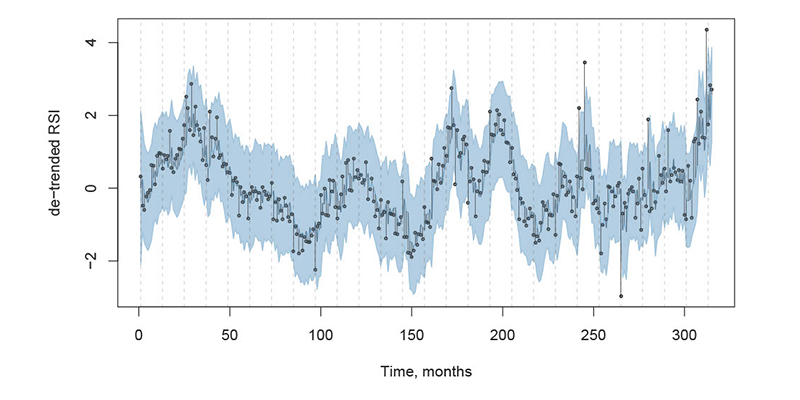
Large-scale data analytics
Large scale data are prevalent in economic and financial research due to advancements in technology and the increasing digitization of financial markets.
For instance, in high-frequency trading, millions of trades on a large number of assets can occur within a single trading day, generating vast quantities of data that can be analysed for insights.
While large-scale data sets are a rich source of information, they also present significant challenges when it comes to extracting reliable trends and predictions. One of the main challenges is the sheer number of variables, which would make a naive model too complex and essentially useless. The temporal dependence among the data should also be properly accounted for. Additionally, the data may contain outliers, which can further complicate the analysis.
To meet these challenges, modern statisticians employ a range of theoretical and computational techniques from a multitude of fields such as statistics, probability, optimization, and machine learning. These techniques allow researchers to process large data sets more effectively, identifying patterns and relationships that might otherwise be hidden or mis-interpreted.
The particular strengths of this research group at York include longitudinal data, functional data, large covariance matrix estimation, nonparametric and semiparametric methods, and robust techniques (in particular rank-based and copula methods).